So says Bernstein.
Did DeepSeek really “build OpenAI for $5M? There are actually two model families in discussion. The first family is DeepSeek-V3, a Mixture-of-Experts (MoE) large language model which, through a number of optimizations and clever techniques can provide similar or better performance vs other large foundational models but requires a small fraction of the compute resources to train. DeepSeek actually used a cluster of 2048 NVIDIA H800 GPUs training for ~2 months (a total of -2.7M GPU hours for pre-training and -2.8M GPU hours including post-training). The oft-quoted “$5M” number is calculated by assuming a $2/GPU hour rental price for this infrastructure which is fine, but not really what they did, and does not include all the other costs associated with prior research and experiments on architectures, algorithms, or data. The second family is DeepSeek R1, which uses Reinforcement Learning (RL) and other innovations applied to the V3 base model to greatly improve performance in reasoning, competing favorably with OpenAI’s o1 reasoning model and others (it is this model that seems to be causing most of the angst as a result). DeepSeek’s R1 paper did not quantify the additional resources that were required to develop the R1 model (presumably they were substantial as well).
But are DeepSeek’s models good? Absolutely – V3 utilizes a Mixture-of-Experts model (an architecture that combines a number of smaller models working together) with 671B total parameters, and with 37B active at any one time. This architecture is coupled with a number of other innovations including Multi-Head Latent Attention (MHLA, which substantially reduces required cache sizes and memory usage), mixed-precision training using FP8 computation (with lower-precision enabling better performance), an optimized memory footprint, and a post-training phase among others. And the model looks really good, in fact it performs as good or better on numerous language, coding, and math benchmarks than other large models while requiring a fraction of the compute resources to train. For example, V3 required -2.7M GPU hours (~2 months on DeepSeek’s cluster of 2,048 H800 GPUs) to pre-train, only ~9% of the compute required to pre-train the open- source similarly-sized LLaMA 405B model (Exhibit 1) while ultimately producing as good or (in most cases) better performance on a variety of benchmarks (Exhibit 2).
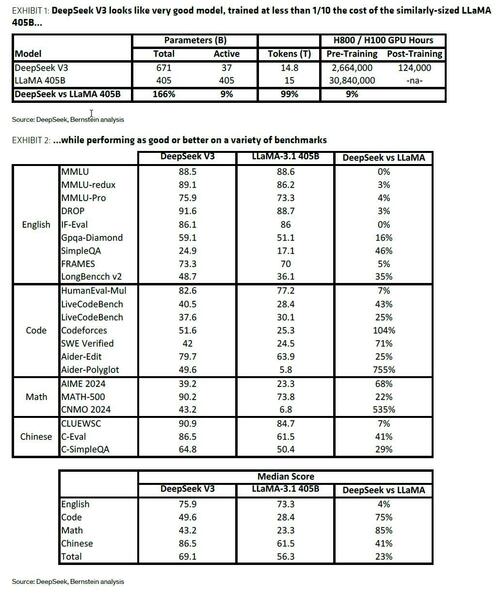